Although the machine-learning discipline is more than 30 years old, the nascent technology is poised for a serious growth spurt that may put puberty to shame.
“As machine learning becomes more mainstream and there’s more understanding of how the technology works, I thing we will see exponential growth,” said Drew Warren, president and CEO of smart-data processing vendor Recognos Financial. “As organizations’ demand increases and grows more powerful, we see more organizations allotting more money to bring its development to the next level.”
Warren attributes machine learning’s increased pace of evolution to better and more abundant enabling technologies, improved performance, and the rise of more specialized vendors that have the potential to reconfigure the technology’s linear improvement curve into an exponential one.
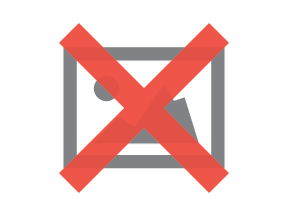
Drew Warren,
Recognos Financial
The advent of open-source resources like Google’s TensorFlow machine-learning library has made development much easier, noted Nitin Rakesh, president and CEO of technology and knowledge-processing outsourcing provider Syntel.
“The in-memory analytical capability is now very powerful,” he said. “More importantly, the collective wisdom that is going into the open-source libraries is very powerful.”
Supplementing machine learning, also known as artificial intelligence, with human wisdom in its production as well as in its development has boosted performance, according to Warren.
“As the technology learns, it makes adjustments, and, in theory, the next time it encounters something similar it should produce a better result,” he explained.”It has been proven that machine learning alone can achieve between 70% and 80% accuracy. When you add humans in to the loop, it aids the learning process and its accuracy rates get much higher — up to a 95% to 98% accuracy rate.”
Finally, most machine-learning vendors have stopped trying to be all things to all people as they did in the 1990s, when the discipline was referred to as semantic technology. Instead, there’s a focus on supporting one of machine learning’s three core benefits — structuring unstructured data, integrating data more efficiently, and improving analytics, according to Warren.
Structuring unstructured data should not be confused with semantic sentiment, in which an engine tags a news story as positive or negative.
That’s easy and trivial, according to Warren. “It really means having computers consume information the way that humans do, by reading,” he said.
Most machine-learning implementations read at about a fifth-grade reading level, but that will improve greatly, according to Warren. “Eventually computers should be able to read at a college level and do some really remarkable things like looking at and interpreting documents.”
As an example, Recognos has implemented an instance of machine learning that lets users pose questions to documents.
“Say that you have an auto insurance policy and a stone cracks your windshield,” he said. “You could look at your policy and ask it if you were covered for the damage. The document would look for the answer in the body of your policy, highlight it, and then present the answer to you.”
Analytics is machine learning’s sweet spot, whether it’s predictive analytics, business intelligence, or progressive analytics.
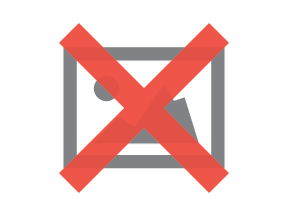
Nitin Rakesh, Syntel
Syntel’s first deployment of machine learning was for predictive maintenance in its production-support environment.
The company’s platform looks at looks at 10 years’ worth of job tickets for applications and looks for signals that may show applications are about to go down and that the IT organization needs to remediate it and bring the application back up.
“It’s a very high-volume and highly resilient application that ‘prophecies’ millions, if not billions, of connections per day,” said Rakesh.
Using this ability to examine outstanding trades for trade breaks would not be that great of a stretch for the technology he added.
“If the machine starts learning to resolve trade breaks based on the nature of the break, it would have a big impact on processing times and the cost of running it in real time, especially when the industry goes from T+3 settlement to T+2 and eventually to T+1,” said Rakesh.
To be sure, not everyone is bullish on artificial intelligence’s potential to transform financial services. Last week at the Futures Industry Association conference in Boca Raton, Florida, Virtu Financial Chief Executive Officer Doug Cifu expressed skepticism regarding the utility of AI, noting that a thinking human must be behind all institutional trading processes.
Featured image by dpullman/Adobe Stock