Artificial Intelligence and Investment Research – Exciting Times Ahead
By Bhawana Khurana, VP Client Solutions for Financial Services at The Smart Cube
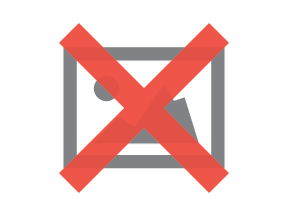
A decade ago, a financial analyst could know almost all that was needed to produce quality investment research; however, that isn’t the case anymore. Today, investing is all about information management, unconventional analysis, and the speed of execution – all at minimal cost. The cost factor has been driving money away from actively managed funds to index funds (passive investing), putting increased focus on stock picking decisions. This is also forcing buy-side professionals to alter the way they conduct investment research.
On the other hand, the sell-side community is dealing with its own set of challenges. A tightening regulatory regime (such as MiFID II) is forcing investment research providers toward a system of unbundling payments from trading commissions and migrating from an unpriced to a priced environment (with direct implication on buy-side firms’ profit margins), i.e., essentially, re-inventing themselves.
Old Hat or Trendsetter?
While the investment research space is witnessing structural changes, both providers and consumers of research are opening up to new ideas, tools, techniques, and thinking. Widespread use of big data analytics and machine learning (ML) to generate and consume investment research is one such idea that has been making some noise for a while now.
However, it is not a new concept – firms have been using neural networks and artificial intelligence (AI) to look for patterns in the FX markets since the 90s. But what needs to be taken into consideration is that back then, the thought process was regarded as, to put it kindly, quite odd.
History has time and again taught us that you can’t hold back an inquisitive mind or ignore any technique/science that can potentially produce an alpha-generating idea. But before we get into the details of how big data and ML actually add value, it is important to understand what these really imply.
What Does it Mean in Theory?
The mainstream definition of big data states that it is a combination of 3Vs – Volume, Velocity, and Variety. Volume refers to the huge mass of data collected from various sources, velocity denotes the speed at which data is processed, and variety describes the different formats in which data is available. A combination of the 3Vs makes it difficult for a single commodity computer to process data, and when that happens, the data is referred to as big data.
Most companies have the capability to collect and store data from multiple sources – with new sources emerging almost every day, such as transactions, social media, and sensors; however, they lag in how to generate any value from it.
The term big data is also used for big data analysis, which is the key to extracting relevant/actionable information from this enormous amount of data. Semantic technology, which provides meaning and context to data, and establishes the relationship between pieces of information, further helps to derive value from large volumes of unstructured data.
And then there is ML, a subset of AI. It basically allows computers to learn from experiences, without explicitly programming them. ML can be supervised or unsupervised – the former is not very scalable, as it requires significant ‘human supervision’, and fails the purpose. While supervised learning mostly deals with problems of classifications and regression, unsupervised learning is favored in case of clustering, i.e., drawing inferences from data sets that comprise input data without labeled responses.
For money managers attempting to navigate the chaos of financial markets, ML is both enticing and promising. Once you start looking into its potential advantages and disadvantages, you realize that there is a whole world of untapped resources at your disposal.
The Sell-side Story
Investment research provider Morningstar, for example, now uses a quantitative equity rating framework generated by an ML statistical model that tries to replicate the results produced by a human analyst. The ML algorithm – Random Forest – has increased the company’s coverage to 50,000 companies that trade on 64 exchanges in 86 countries.
Hence, for the sell side, a wider adoption of big data analytics and ML can lead to the following:
- Faster and Lower Cost Research – Companies can avoid major technology investments by leveraging the scale and processing power of cloud-based infrastructure and analytics software. They can collect, correlate, and analyze huge, complex data sets and build models in a fraction of the time and cost that it takes in-house analysts.
- Increased Accuracy of Research – ML and advanced predictive analytics techniques can be potentially far more reliable and scalable than models built in MS Excel spreadsheets. In addition, patterns can be detected to capture small nuances in stocks/markets/
economies and build robust research models. - Improved Client Service – ML software can make sell-side companies more competitive. These can be leveraged to improve client services by making select tools available to individual investors and justify the value of research provided to each client.
Buy-side Performance
Similarly, these tools and techniques are opening up new possibilities for buy-side players. ML and AI can automate investment management activities – such as research and portfolio construction – and the use of open-cloud services can transform their cost structure.
Asset managers can leverage the power of data analytics to adapt to changing investor, regulatory, and competitive dynamics in the following ways:
- Trade Signals and Automated Trading: Predictive analytics – using statistics, data analysis, and modelling to identify patterns and forecast performance – sentiment analysis and news analytics, along with language recognition algorithms (to quantify news items and assessing the positive and negative relevance), can all be used to automate trade signals and trading strategies.
For example, at TSC, we automatically analyze multiple news feeds from various sources and provide curated alerts to clients at per the requisite frequency and sensitivity.
- Improved Fund Performance via Alternative Data: Alternative data refers to any data that is traditionally not considered financial data, but may offer investors market insights. For instance, social media, GPS, and spatial data can be layered into financial models using techniques such as text-mining analytics or natural language processing (NLP) and can significantly improve the level of insights drawn into specific securities/trades, and generate better trade results and fund performance.
- Higher Research Productivity Using Automation: With margins compressing for the buy side (especially for active asset managers), productivity of investment professionals is becoming an increasingly critical element. Significant amount of a research analyst’s time is spent on data gathering and organizing, much of which can be easily automated and analyzed using AI and ML.
- Enhanced Portfolio Risk Management: Tightening regulations and enormous complexity of risk factors have created the need for deeper integration and more powerful detection capabilities to manage portfolio and enterprise-wide risks. Modern risk management requires more than just analysis of past or current events; it requires the anticipation/prediction of events before they actually take place.
The Bottom Line
Scientific Active Equity (SAE) – an investment group at BlackRock, with $8o billion assets under management (AUM) – is one of the players leveraging this new kind of fundamental research. The group uses big data to run detailed diagnostics on thousands of stocks globally and bases the fundamental research on commonly used metrics, such as cash flow or earnings growth. However, instead of meeting management personnel or attending conference calls, SAE relies on machine reading and algorithms to analyze SEC filings, conference calls, news releases, and transcripts from investor days.
This has helped SAE in covering thousands of companies every quarter, as opposed to simply undertaking the exercise for a couple of companies every day. The techniques have also enabled the group to look for inconsistencies in manager comments or spot patterns that may otherwise go unnoticed.
To put it simply, the investment industry is on the cusp of some big structural changes. As the use of ML and AI gains strengths, human asset managers and research analysts are more likely to adapt to the changing environment, as opposed to the rampant fear of simply disappearing. The future of investment management most likely involves a synthesis of human and artificial intelligence that harnesses the power of both. And there definitely are interesting times ahead!